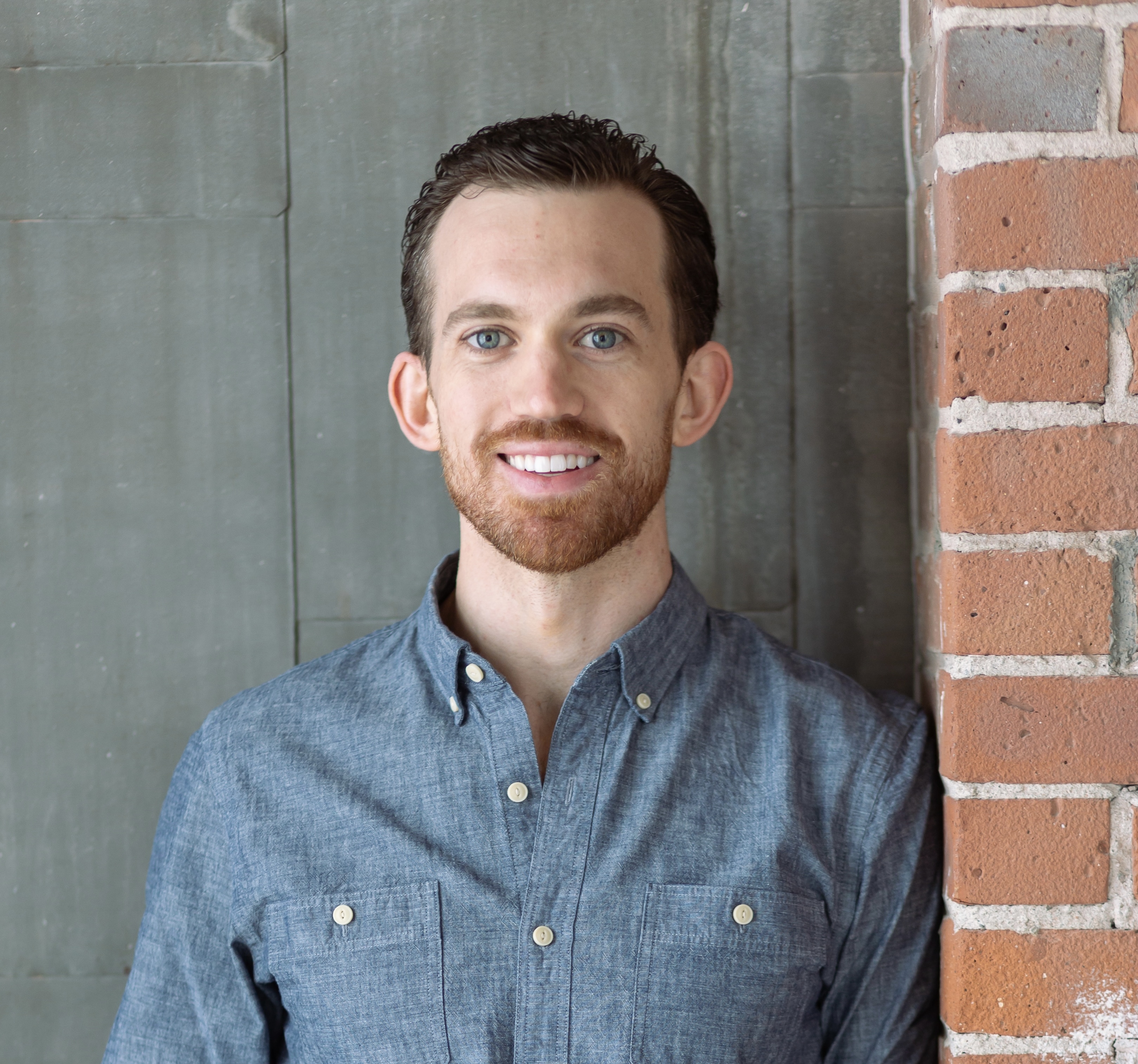
I'm a data scientist and bioinformatician broadly interested in improving human health by extracting actionable information from modern biological and health-related datasets. My contributions to this wide-reaching goal generally include developing reproducible data pipelines, predictive machine learning models, and intuitive visualizations.
I'm currently a Bioinformatics Scientist at the Bill & Melinda Gates Medical Research Institute (opens new window), where I build cloud-based pipelines and machine learning models for next-generation sequencing data in the pursuit of discovering predictive biomarkers to support drug and vaccine clinical trials.
Prior to joining the Gates, I was a Data Scientist on the bioinformatics team at Indigo Agriculture (opens new window), where I developed methods and tools for analyzing microbiome datasets generated by amplicon sequencing experiments.
The primary goal of my work at Indigo was to model the complex relationships between microbe abundances and the health of their host plants using a blend of modern machine learning methods and statistical inference techniques.
Previously, I was a staff scientist in the Neale Lab (opens new window), located at the Analytical and Translational Genetics Unit (ATGU) (opens new window) at Massachusetts General Hospital and the Stanley Center for Psychiatric Research (opens new window) at the Broad Institute of MIT and Harvard.
In the Neale lab, my work focused on using statistical methods and computational techniques to elucidate the genetics underlying human traits and diseases.
I received a Master of Science in Applied Statistics from the University of Michigan and a Bachelor of Arts in Mathematics from Williams College.
If you see any similarities between your work and mine you'd like to discuss, or if you'd just like to introduce yourself, send me a note at liam.e.abbott@gmail.com!